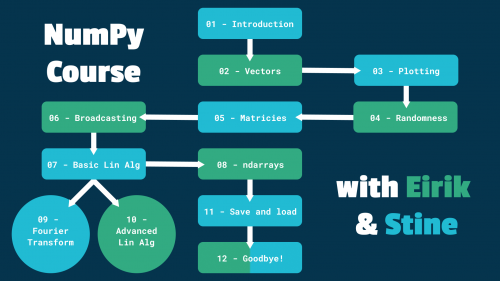
SkillShare – Scientific Computing with NumPy Python Data Science-SkilledHares
English | Size: 1.77 GB
Category: Tutorial
Hi there!
We’re a couple (Eirik and Stine) who love to create high-quality courses!
SkillShare – Scientific Computing with NumPy Python Data Science-SkilledHares
English | Size: 1.77 GB
Category: Tutorial
SkillShare – Introduction to Data Science for Complete Beginners-SkilledHares
English | Size: 1.78 GB
Category: Tutorial
Apress – SQL Server Transparent Data Encryption Protecting Database Files at Rest-iLLiTERATE
English | Size: 31.33 MB
Category: Tutorial
PluralSight – Spring Framework-spring Data Jpa With Hibernate Bookware-KNiSO
English | Size: 469.48 MB
Category: Tutorial
Udemy – GIS Data Analysis with ArcGIS Desktop
English | Size: 2.0GB
Category: Tutorial